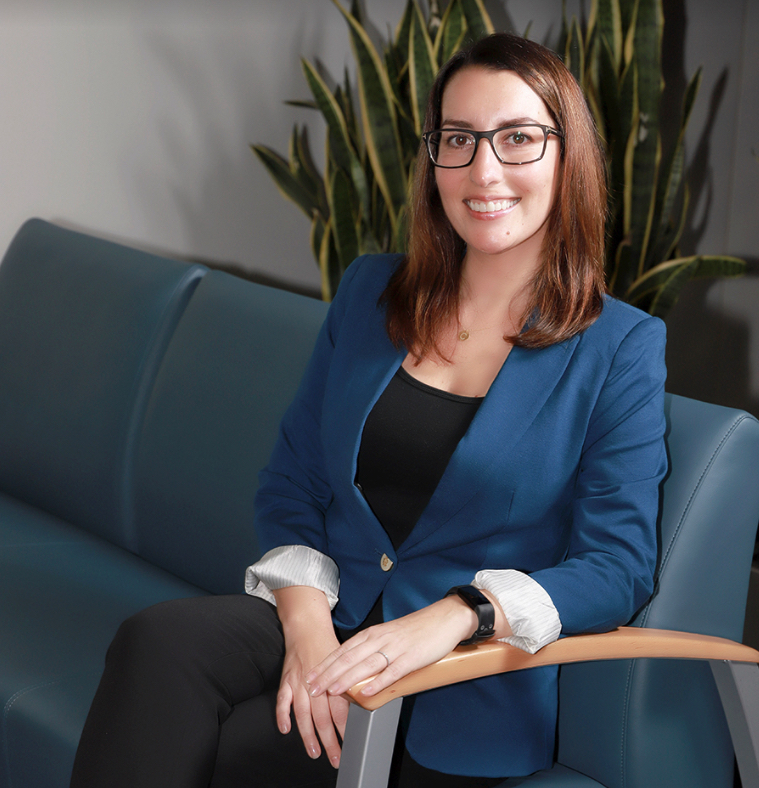

The demand for clinical trial testing is rising, from over 260,000 trials conducted in 2018 to nearly double that figure in 2024. This means that more trials are being conducted simultaneously than ever—a factor that adds to the already challenging process of finding clinical trial participants.
Because trial sponsors are struggling to find sufficient numbers of suitable participants, 80% of the world’s more than 450,000 clinical trials are at risk of delays. Maintaining their participation throughout trial is another challenge all its own. The complexities that come with these two efforts—recruitment and retention—are also the root causes of delays in clinical trial completion.
AI presents an opportunity to reimagine these difficult, lengthy and error-prone processes with a new level of efficiency. But as clinical trial recruiters and managers work to define AI’s role in improving participation and retention, they’re also discovering AI’s limitations. More specifically, they’re realizing where AI’s work should end, and humans’ work should begin.
Our own efforts have proven that ongoing human-to-human interactions are critical to creating the type of positive patient experiences that make-or-break participants’ willingness to consider trials–and stay in those trials for the long haul. By distinguishing between which tasks AI and advanced technologies like machine learning should be involved with, and those that skilled staff should own, we’ve developed a hybridapproach that’s resulted in an up to 50% increase in patient randomizations into clinical trials.
AI finds new potential trial participants.
With multiple studies reaching into the same patient population pools, trial sites are struggling to efficiently enroll similar trials that fall within comparable timeframes. This can even happen when trials generate an overwhelming amount of upfront interest from potential participants.
For example, there may be two obesity trials occurring at the same time, in nearby locations, with very similar participant requirements. With 42% of the adult population in America living with obesity, there is no lack of people who want to get involved with these trials–making it seem like it would be relatively easy to find participants. But the volume of people raising their hands to participate does not eliminate other challenges. Most obesity trials, for instance, include comorbidity criteria such as diabetes, kidney disease, heart disease or fatty liver. This type of criteria inevitably decreases the pool of qualified participants.
For trial sites, filling participant gaps in any trial can require going beyond their relatively comprehensive databases of potential candidates to find new, previously unidentified ones. This is one place where AI can and should step in to do what it does best: distill vast amounts of information into usable insights.
Across trials, there may be many suitable candidates who would qualify for life-changing clinical trials—if only they knew these trials were being conducted or how to get started as a participant. Lack of patient awareness is one of the most significant challenges to identifying and reaching new participants. It’s also a missed opportunity for participants who can gain valuable health benefits as a result of their involvement.
To overcome these challenges, sites rely on outside vendors for recruitment assistance through direct advertising and advocacy efforts. Social media outreach and digital advertising have become key players in reaching those untapped participant populations by meeting them where they already spend time. Now, with AI and machine learning emerging as viable tools, recruitment efforts can leverage historical data from recruitment firms and pharmaceutical companies to more quickly identify potential candidates. And it can do it at a speed and scale that simply wasn’t possible before.
Because AI learns as it goes, it becomes smarter than the original data set it started with. This means that trial sponsors can go beyond simply targeting ads at, say, people within 20 miles of the trial site who meet the trial’s age criteria. While this is no doubt a good and logical place to start, the potential created by using advanced technology is infinitely greater.
The human touch is what turns candidates into full-duration participants.
Once AI has assisted in the critical task of significantly scaling potential participant identification—exponentially faster and more efficiently than humans can achieve on their own—skilled nurses step in to conduct the more extensive health assessments. These screenings are necessary to ensure that candidates fully meet the trial’s protocol, including whether their health conditions or lifestyle factors will ultimately prevent them from participating for the duration of the trial.
In the case of Alzheimer’s Disease research, for instance, while a given patient might be fully qualified and perfectly suited for the trial health-wise, lifestyle factors such as a caregiver’s inability to join alongside them, scheduling conflicts or a lack of transportation can get in the way of participation.
Identifying these types of subtleties falls firmly into the domain of humans. Nurses can navigate these topics with a level of nuance that can’t be achieved by AI’s analysis of online questionnaires or other data processing approaches.
Through the process of having AI handle participant identification and then passing the torch to nurses for further screening, we have seen an increase in patients randomized into trials by as much as 50%.
From there, keeping participants engaged for the duration of trials means providing human-led and patient-centric experiences that prioritize their ongoing satisfaction.
Human care helps to ensure patient-provider trust.
Across industries, organizations are looking to deploy AI in ways that play to the technology’s strengths and free humans to leverage their strengths as well. One of the areas technology falls short in most scenarios is in aiding in uniquely human experiences, especially in a deeply personal and intimate field such as healthcare.
Patients whose conditions are life-threatening or otherwise debilitating may be experiencing confusion or anxiety linked directly to their diagnoses or treatment plans. Well-trained human nurses and clinicians leaning on years of experience make all the difference here in a way today’s AI interfaces simply can’t.
While the technology will undoubtedly continue to evolve, long-term engagement and genuine connection will continue to stem from real human-to-human interaction for the foreseeable future. There is no substitute for a gentle demeanor, an empathetic gesture, or a compassionate gaze when patients might be uncertain, frustrated, or even frightened.
The broader healthcare industry must continue to negotiate the role of AI, but never at the cost of the kind of human care that is a patient’s right. In clinical trials, the technology isn’t ready to be relied on for every key function, and due to the personal nature of healthcare, may never be. There are other considerations that must be weighed, as well, such as patient privacy and data security, and the emergence of AI compliance regulations around the world.
One thing that’s not up for debate is that with the right tools and people doing the right jobs, we’ll be able to bring potentially life-changing treatments to the people who need them most, a lot quicker.
About Cara Brant
Cara Brant is the CEO of Clinical Trial Media, a global clinical trial participant recruitment and retention company that works with pharmaceutical companies through all stages of clinical research. She has held the position since 2018, when she purchased the company after previously serving as its COO. A graduate of Ithaca College, Brant is dedicated to improving site and patient journeys through data-driven innovative solutions.