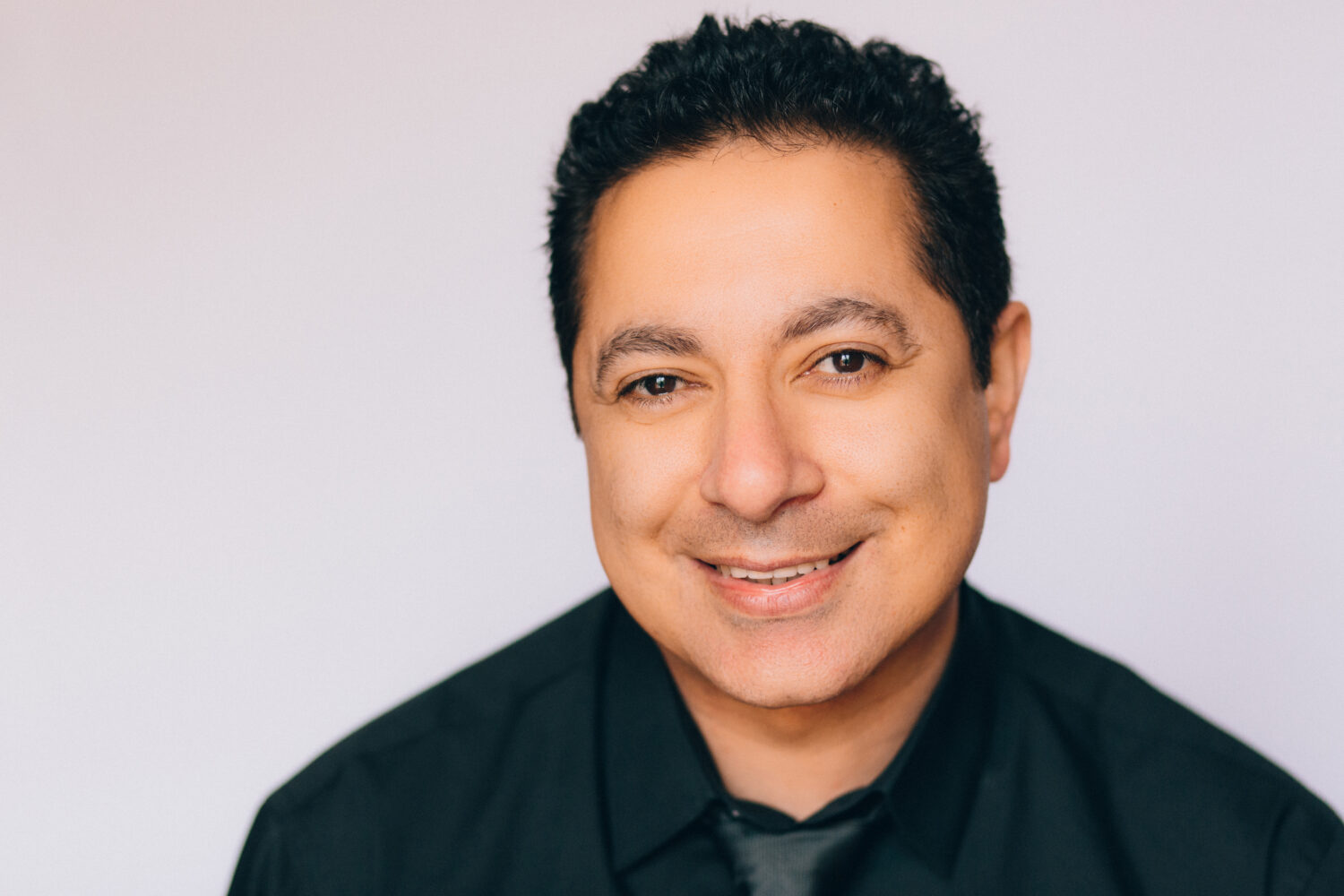

McKinsey & Company estimates it takes up to 12 years to introduce a new drug to the market – a lengthy timeline comprised of many factors including technology limitations, regulatory requirements, and patient recruitment and retention. Scientific innovation and clinical trials are increasingly complex, generating more data than ever. Researchers are facing difficulties managing the volume and variety of data, turning to artificial intelligence, machine learning and advanced data architecture to improve data processing, user experiences, and outcomes.
However, one less discussed challenge within the drug discovery and development lifecycle is the ability of compute power to not only advance the data infrastructure needed but to also meet the increasing data demands of new and complex clinical trials. Building on tech advancements of the past decade, breakthroughs in technological hardware are poised to exponentially increase the capabilities of the compute, storage, and transfer of data. Continued momentum will enable future techniques that are not feasible today across mainstream industries, including drug discovery and clinical research.
Supercomputer and Quantum Computing Possibilities in Clinical Trials
Recent developments in compute power can help researchers solve problems that are too complex for classical computing. The May 2022 introduction of the National Laboratory’s Frontier, currently considered the fastest supercomputer in the world, was groundbreaking for the scientific and research community. Operating with a performance of 1.1 exaflops, this supercomputer technology has been responsible for processing data at unbelievable speeds (one quintillion calculations per second). This is monumental for the life sciences industry, enabling scientific teams to process large quantities of data and test new discoveries faster. Still, by contrast, a quantum computer can solve a complex mathematical problem (such as Shor’s algorithm) millions of times faster than the fastest supercomputer in existence today.
Over recent years, we’ve seen many breakthroughs on the quantum computing front, opening the door for a hybrid compute approach of unprecedented speed and complexity. This includes the discovery by IBM in quantum computing, published by the scientific journal Nature as it relates to noise reduction and error mitigation in quantum qubits. IBM ultimately solved a complex problem that leading supercomputing approximation methods could not handle for years using the IBM Quantum ‘Eagle’ quantum processor (with the power of 127 superconducting qubits on a chip), allowing the team to generate massive amounts of power that simulated the dynamics of spins to precisely predict properties such as its magnetization. Even more recently, we’ve seen technology giants like Microsoft and Quantinuum announce a breakthrough in the quantum field. Through applying Microsoft’s error-correction algorithm to Quantinuum’s physical qubits, the two achieved a record of logical circuit error rates 800 times fewer than its corresponding physical circuit error rates.
These achievements accelerate the timeline towards a future in which researchers could leverage these high-performing systems to solve previously intractable problems involving trillions of data points, such as molecular and atom simulations.
Continued Advancements in Storage and Data Transfer Capabilities
In a study published by The National Library of Medicine (NIH), researchers employed a machine learning algorithm to analyze data from over 16,000 clinical trials and discovered that the average complexity score across all trials surged by over 10 percentage points in the last decade. In addition to trials becoming more complex, it’s evident that today’s clinical trial teams are grappling with an unprecedented volume of data. In 2021, Tufts Center for Study of Drug Development (CSDD) found that phase III clinical trials produced 300% more data points in the last decade, collecting an average of over 3.6 million data points.
To cope with this data overload, life sciences companies are seeking ways to automate digital data
flows, from ingestion to analytics, to expedite data cleaning, and decision-making processes, ultimately facilitating faster insights. This is where leveraging the power of data architecture comes into play. Data architecture is often underestimated, but it can establish standardized procedures for capturing, storing, transforming, and delivering actionable data for its users.
However, for clinical researchers to harness the full power of data architecture to extract meaningful insights, the capabilities of its data storage and data transfer need to be advanced enough to process, manage, and centralize large volumes of data—often coming from different sources. Processing capabilities were limited by classical computing systems, but in recent years we have seen major developments on the data storage and data transfer side that have advanced the evolution of data architecture.
In the area of data storage, a team of scientists at the University of Rochester led by assistant professor Stephen M. Wu developed hybrid phase-change memristors that offer super-fast, low-power, and high-density computer memory. Advancements such as these can generate computer memory that is ultra-fast and efficient, expanding possibilities for the data volume that can be stored, accessed and utilized in clinical trials.
On the data transfer side, researchers at Technical University of Denmark in Copenhagen developed a single computer chip that transferred 1.84 petabits of data per second – that is equivalent to downloading over 200,000,000 pictures in one second. To put that in the context of clinical research, a standard trial today generates under 1 terabyte of data from beginning to end, an amount that can be transferred in a couple of hours over a standard connection. In precision medicine, where genomics data is captured and stored in variations per patient, a medium-sized trial can generate petabytes of data – with 1 petabyte requiring 90 days for a standard connection data transfer. As these hardware advancements continue to remove the technological hurdles of data storage and processing it will unleash the potential of what can be done with the data of personalized medicine.
Unlocking New Breakthroughs in Drug Discovery
Technological breakthroughs will play a pivotal role in revolutionizing the most complex and data-driven areas of the drug discovery process, including molecular dynamic simulations, quantum chemistry calculations, genomic and bioinformatics, AI and more. Recent innovations are already transforming drug discovery by offering unprecedented capabilities that can identify potential drug targets and result in novel therapeutics. As we continue to see the advancement of computing, data transfer, and storage power, we will increase the access to answers in these volumes of data, allowing researchers to discover new therapies and speed up timelines, getting treatments to patients sooner.
About Sam Anwar
Sam Anwar is the Chief Technology Officer at eClinical Solutions where he harnesses the power of software development, big data, AI and machine learning to help advance technology innovation in life sciences.Sam has spent the past 20 years of his career leveraging cutting edge technologies to revolutionize clinical trials. In addition to software development, Sam has deep expertise in a diverse set of technologies including IT infrastructure, web technologies, information security, database design, business intelligence, big data platforms and analytics.