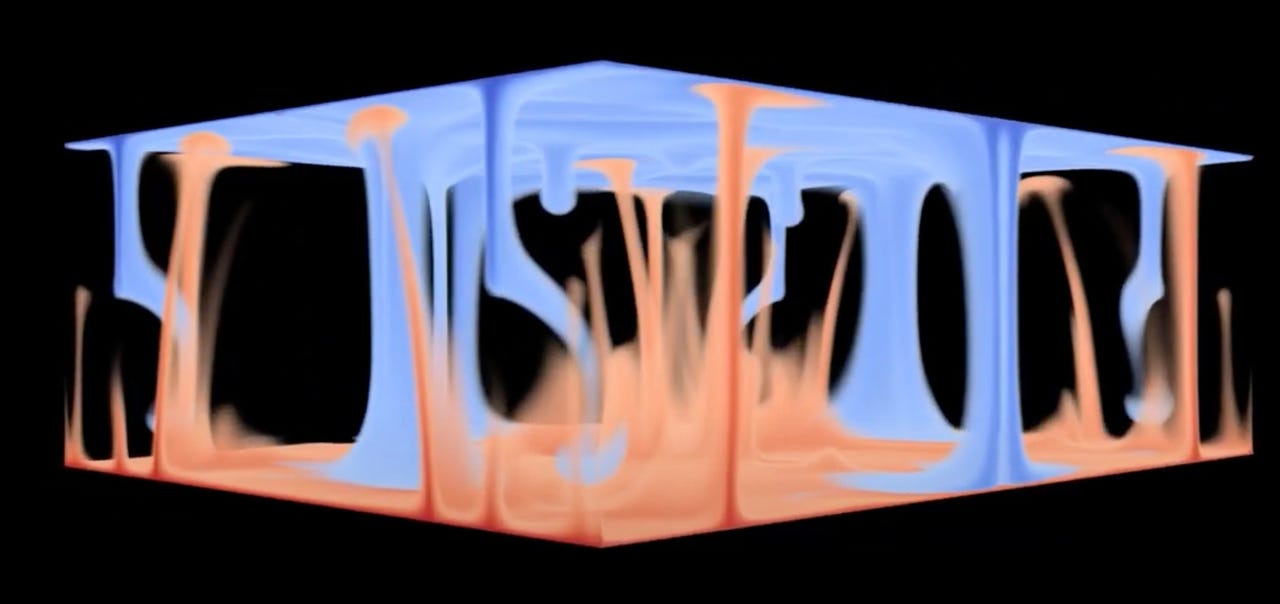
Computer maker Cerebras used its AI computer on a non-AI problem: simulating “buoyancy-driven Navier-Stokes flows” that capture dynamics of many systems in nature and the built environment. The work, the first of its kind, allows for a “digital twin” of the real-world whereby scientists can make predictions and see the effects of interventions in a kind of control loop. Cerebras/DoE NETL 2023
Simulating the real world in real time can afford scientists a way to make predictions based on playing out scenarios as they unfold. That could be an asset in dealing with extreme weather scenarios, such as those involved in global warming.
AI computing pioneer Cerebras and the National Energy Technology Laboratory of the U.S. Department of Energy on Tuesday announced a speed-up in scientific equations that they say can permit real-time simulation of extreme weather conditions.
Also: Fighting climate change: These 5 technologies are our best weapons
“This is a real-time simulation of the behavior of fluids with different volumes in a dynamic environment,” said Cerebras CEO Andrew Feldman.
“In real time, or faster, you can predict the future,” Feldman said. “From a starting point, the actual phenomena unfolds slower than your simulation, and you can go back in and make adjustments.”
That kind of simulation, a digital twin of real-world conditions, essentially, allows for a “control loop” that will let one manipulate reality, said Feldman.
In prepared remarks, Dr. Brian J. Anderson, lab director at NETL, said, “We’re thrilled by the potential of this real-time natural convection simulation, as it means we can dramatically accelerate and improve the design process for some really big projects that are vital to mitigate climate change and enable a secure energy future — projects like carbon sequestration and blue hydrogen production.”
Anderson added, “Running on a conventional supercomputer, this workload is several hundred times slower, which eliminates the possibility of real-time rates or extremely high-resolution flows.”
In a video prepared by the researchers, streams of hot and cold fluids flow up and down like an alien landscape.
Cerebras has made a name for itself with exotic hardware and software for large artificial intelligence training programs. However, the company has added to its repertoire by targeting challenging problems in basic science that are compute-intensive that may have nothing to do with AI.
In the domain of computational fluid dynamics, the Cerebras machine, called a CS-2, is able to simulate what is known as Rayleigh-Bénard convection, a phenomenon that results from a fluid being heated from the bottom and cooled from the top.
Also: Data and digital twins are changing golf. They might fix your golf swing, too
The work was made possible by running a new software package developed last fall by Cerebras and NETL called the WSE field equation API, a Python-based front end that describes field equations. Field equations are a type of differential equations that “describe almost every physical phenomenon in nature at the finest space-time scales,” according to the GitHub documentation.
Basically, field equations will model everything in the known universe other than quantum entanglement.
The API, described in the November paper “Disruptive Changes in Field Equation Modeling: A Simple Interface for Wafer Scale Engines,” posted on arXiv, was designed explicitly to take advantage of the Cerebras computer’s special AI chip. The chip, called the Wafer Scale Engine, or WSE, debuted in 2019 and is the world’s largest computer chip, the size of almost an entire semiconductor wafer.
The paper in November described the WSE as able to perform field equations two orders of magnitude faster than NETL’s Joule 2.0 supercomputer, built by Hewlett Packard Enterprise using 86,400 Intel Xeon processor cores and 200 of Nvidia’s GPU chips.
Because of their highly distributed nature, supercomputers are prized for their ability to run components of equations simultaneously in order to speed up the total computation time. However, the NETL scientists found that running field equations runs into bandwidth and latency limitations of moving data from off-chip memory to the processor and GPU cores.
Also: AI startup Cerebras celebrated for chip triumph where others tried and failed
The field equation API instead made use of the ability of the Cerebras WSE’s vast on-chip memory. WSE 2, the second version of the chip, contains 40GB of on-chip memory, as thousand times as much as Nvidia’s A100 GPU chip, the current mainstream offering from Nvidia.
As the NETL and Cerebras authors describe the matter,
While GPU bandwidth is high, the latency is also high. Little’s law dictates that a large amount of data needs to be in flight to keep utilization high when both latency and bandwidth are high. Sustaining significant amounts of data in flight translates to large subdomain sizes. These single device scaling properties, limit attainable iteration rates on GPUs. On the other hand, the WSE has L1 cache bandwidths and single cycle latency, thus the attainable iteration rates on each processor are much higher.
The simulation operates on a kind of Excel spreadsheet of over 2 million cells with values that change.
While the research in November found the CS-2 to be far faster than the Joule at field equations, the scientists at NETL have not yet reported official speed comparisons for the fluid dynamics work announced Tuesday. That work is in the process of being undertaken on a cluster of GPUs for comparison, Feldman said.
Said NETL and Cerebras in the press release, “The simulation is expected to run several hundred times faster than what is possible on traditional distributed computers, as has been previously demonstrated with similar workloads.”
Also: I asked ChatGPT to write a WordPress plugin I needed. It did, in less than 5 minutes
The Cerebras CS-2 machine used in the project is installed at Carnegie Mellon University’s Pittsburgh Supercomputing Center as part of the Neocortex system, a “high-performance artificial intelligence (AI) computer” funded by the National Science Foundation.
The current work is not the first time that Cerebras has branched out from AI. In 2020, in another partnership with the DoE, the WSE chip excelled at another problem set of partial differential equations in fluid dynamics.
Cerebras CEO Feldman indicated there will be lots more opportunities for the company in the area of scientific computing.
The simulation of buoyancy-driven Navier-Stokes flows, noted Feldman, takes advantage of “foundational” equations in computational fluid dynamics.
“The fact that we can crush it in this simulation bodes very well for us across a wide swath of applications.”